PhD Students

Zachary Althof
Zac Althof studies the fissures that divide the lobes of the lung through deep learning. His work has developed an automated method for assessing the completeness of these fissures (degree to which they separate the lobes in the lung). Because a lack of fissure completeness has been shown to be a biomarker of airflow between lobes, assessing fissure completeness from CT images offers a non-invasive method to screen for treatments that aim to improve patient quality of life through blocking airflow to diseased regions. The next step in his PhD is deploying this automated method on large cohorts of data, seeking to understand fissure completeness trends within the population and its link to pulmonary biomechanics and chronic diseases such as COPD.

Joshua Carrizales
Joshua Carrizales works on improving radiation therapy for lung cancer patients using artificial intelligence. His work focuses on correcting corrupted four-dimensional computed tomography (4DCT) images that are commonly used to plan radiation therapy. His work was well received at the 2022 National GEM Consortium conference as he was awarded 1st place in the technical presentation competition for the early PhD group. Moving forward in his PhD, Joshua hopes to develop automated tools to help in the treatment of cancer patients.
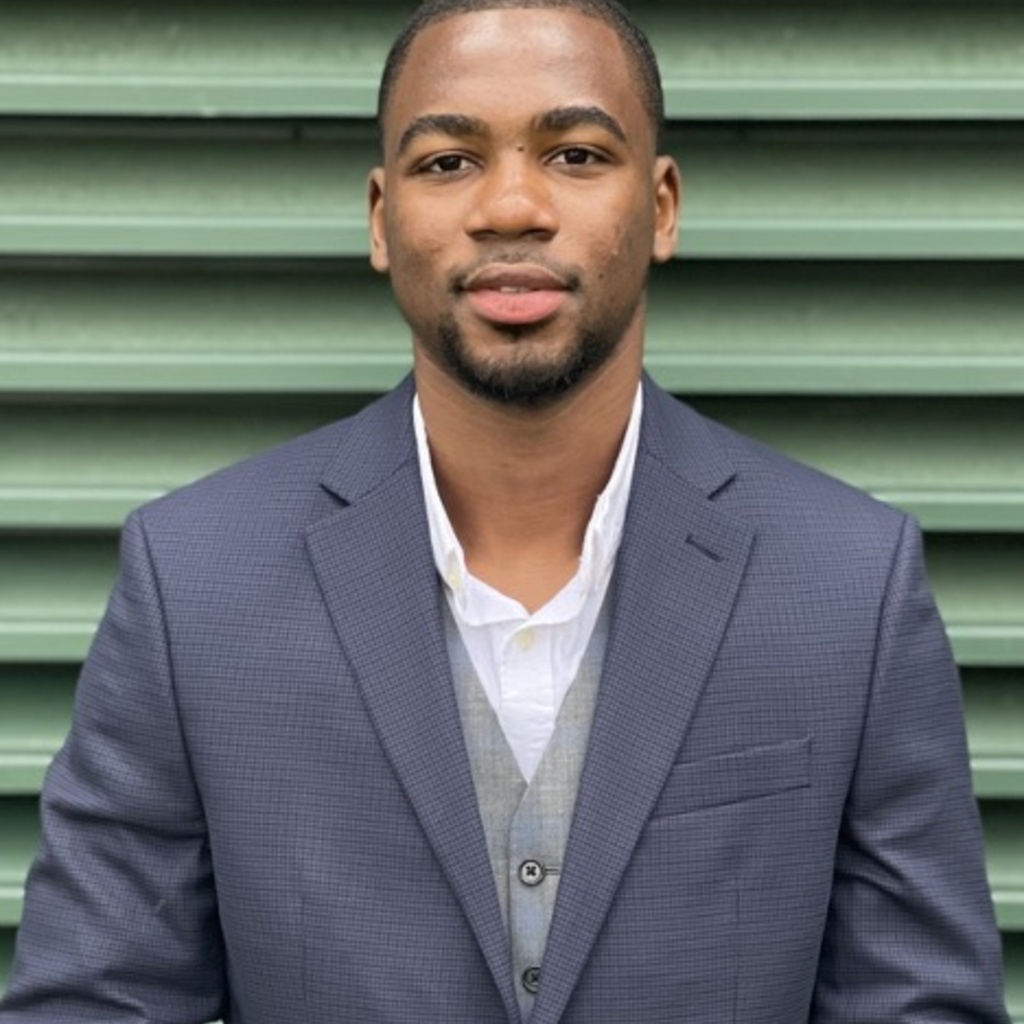
Akor Emmanuel
Acute Respiratory Distress Syndrome (ARDS) is a type of respiratory failure characterized by the heterogeneity of regional mechanical properties of the injured lungs. Currently, no drug has proven to be effective in managing ARDS, hence intubation and mechanical ventilation is the support mechanism used in addressing this pathophysiology. Simulating the mechanical ventilatory-induced lung behavior in-silico using computational methods is ideal to allow for varying frequencies and tidal-volume combinations to be assessed for each ventilation type. Hence, my research objective is to investigate the structure-function changes of the ARDS lung under mechanical ventilation using computed tomographic images. This objective will be met by developing patient-specific forward computational models that can predict ventilation, airflow distribution, and tissue structure deformation.

Adam Galloy
Title/Position
Graduate Research Assistant
Mentor: Dr. M.L. Suresh Raghavan
Lung mechanics can provide a scientific basis for developing new tools to diagnose, characterize, and treat lung diseases. One often overlooked aspect of lung mechanics is the sliding motion between the five lobes of the lungs. Although most people have complete lobe boundaries that allow for sliding between lung lobes, some people may have incomplete or deteriorated lobe boundaries that can prevent sliding and affect breathing mechanics. The goal of my research is to improve our understanding of how lobar sliding (or the lack thereof) affects the mechanics of breathing. To achieve this goal, I use computational mechanics and medical image processing techniques such as finite element analysis and deformable image registration to model and characterize lobar sliding and its impact on breathing.

Kevin Knoernschild
My research is focused on training machine learning classification models for lung cancer risk prediction, as well as rapid Chronic Obstructive Pulmonary Disease (COPD) progression prediction. My current role is data preprocessing and standardization of thousands of low dose computed tomography (LDCT) lung images from the National Lung Screening Trial (NLST). Additionally, I am developing a semi-automated pulmonary nodule segmentation method for the NLST cohort, which contains a large range of different image reconstructions, pulmonary nodule types, and pulmonary nodule sizes. This image standardization and pulmonary segmentation work is in preparation for quantitative radiomic feature extraction of the pulmonary nodules, which will be used alongside DNA methylation data as the basis for training our machine learning models for lung cancer risk prediction, as well as whole lung rapid COPD progression prediction.
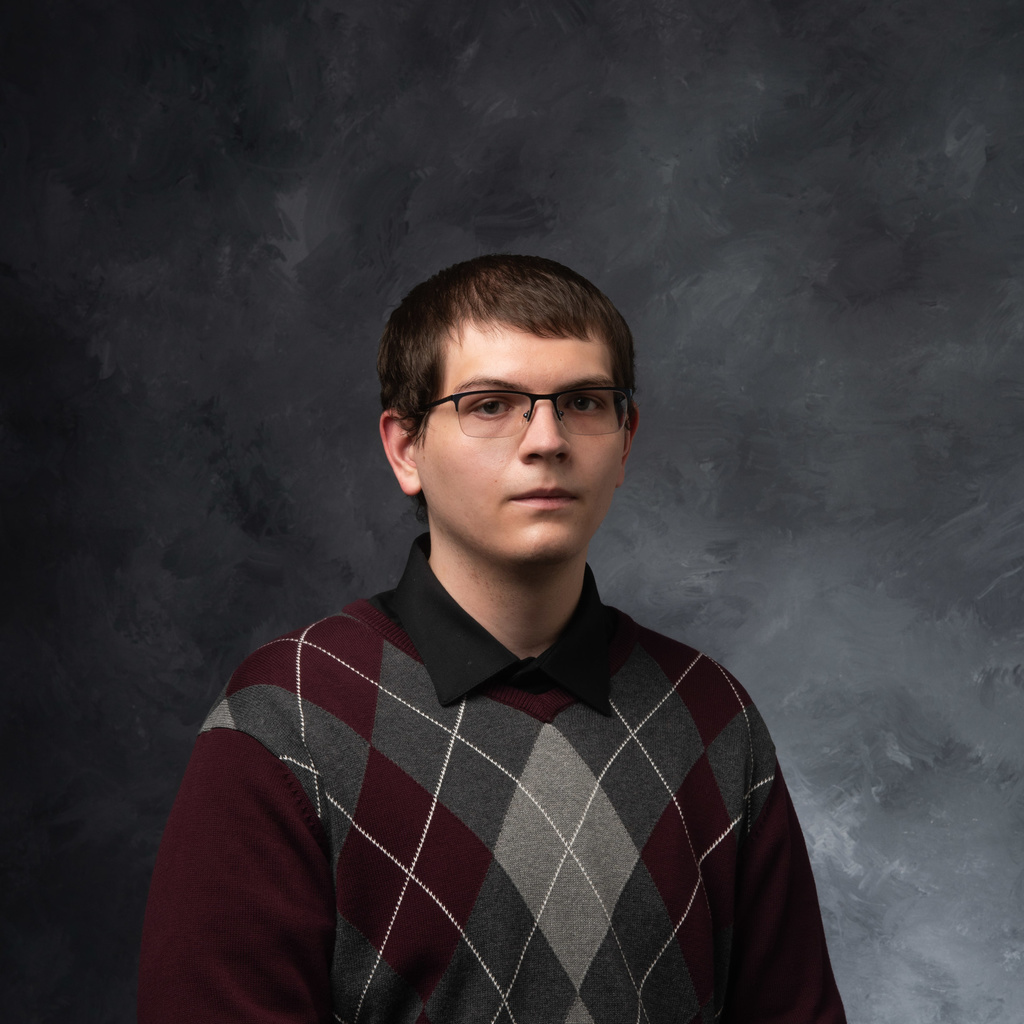
Max Sampson
Mentor: Dr. Kung-Sik Chan;
I am a PhD student in the statistics department at the University of Iowa. I work on statistical analyses to see how smokers can decrease inflammation in their lungs through the restoration of perfusion in areas of smoking associated infiltrates. My focus is on taking into account the bias that can exist in randomized controlled trials due to missing data from dropouts. This bias can hide practically important findings as well as remove an ability to make cause and effect statements about the findings.

Qi Wang
Mentor: Dr. Kristan Worthington
Qi Wang is an MD-PhD trainee at University of Iowa Carver College of Medicine’s Medical Scientist Training Program. Her current research involves utilizing photopolymerizable biomaterial to develop an in vitro model for studying pulmonary fibrosis. Photopolymerizable biomaterials are versatile and relatively easy to manipulate to control chemistries and stiffnesses. Thus, they are excellent substrates for studying lung cell behavior as they can be used to mimic stiffness, chemistry and cyclical strain experienced during healthy and pathological conditions. By observing cell behavior on our in vitro lung system using microscopy, we can gain an understanding of how fibrosis progress given specific substrate chemistry and mechanical stimuli.
Alumni
Erik Cole
Title/Position
Graduate Research Assistant
Mentor: Dr. Joseph Reinhardt
Identifying COPD severity and predicting COPD progression from CT images can help identify the way in which an individual is affected by COPD. The structure of the airways (airway size, length, branching angle) is not commonly studied in relation to disease progression. We apply machine learning techniques to study the airways that are segmented from the CT images. Through unsupervised learning techniques such as clustering trends can emerge. In addition, I am utilizing graph neural networks to allow for more complicated comparisons to be made. In the future this will allow us to define COPD phenotypes and broadly classify how the disease progresses over time.
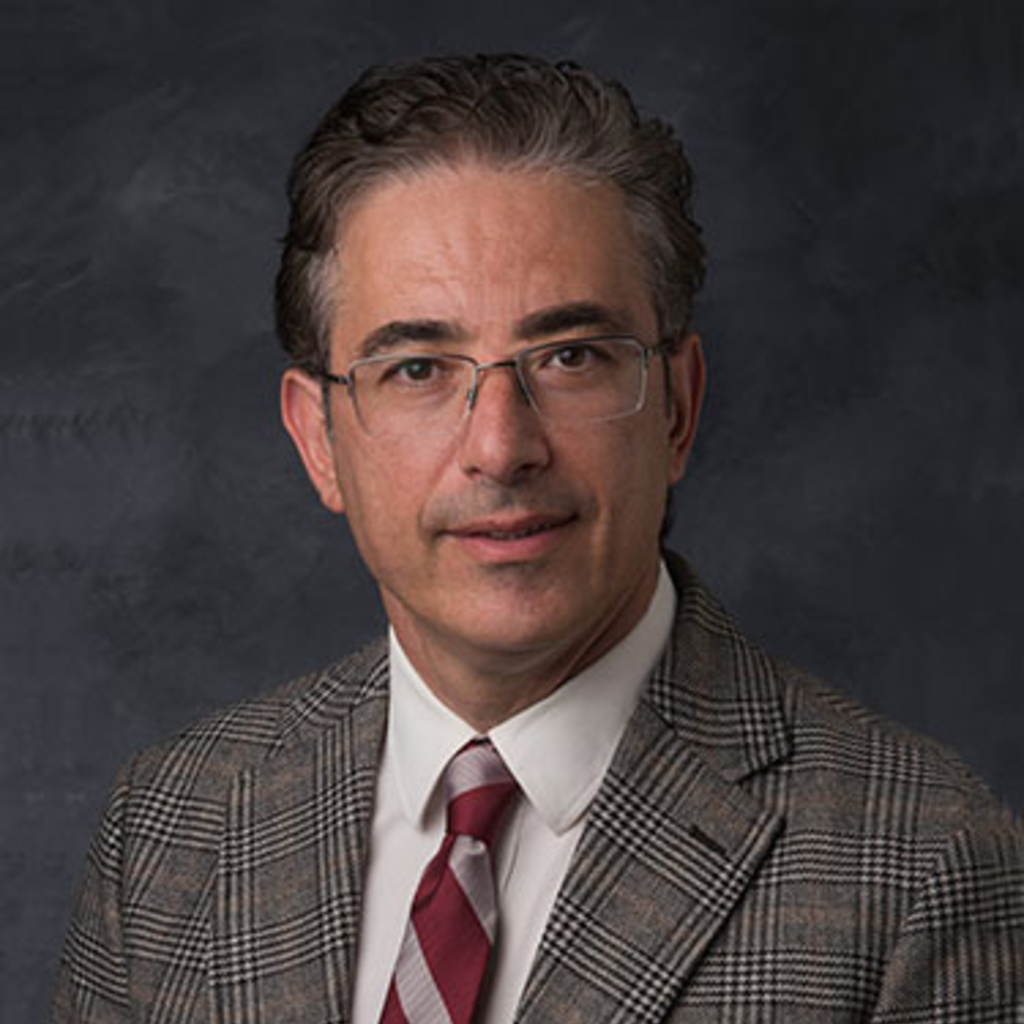
Ali Eskandari
Title/Position
Postdoctoral Research Fellow
I use dual-energy CT to assess pulmonary parenchymal perfusion characteristics in a large multi-center study (MESA Lung) serving to phenotype risks for chronic obstructive pulmonary disease. Our working hypothesis is that individuals shutting down perfusion in inflamed parenchymal regions will be most susceptible to the development of emphysema. My role has been evaluating the images of 750 volunteers, developing metrics serving to characterize both the scan characteristics as well as to characterize the pulmonary pathologic indices. My experience with DECT of the lung has extended to evaluating similar scans of patients being evaluated for pulmonary emboli with a diagnosis of COVID-19, and utilizing our texture analysis software to evaluate non-contrast scans of COVID-19 patients for signs which seem to indicate micro-embolic disorders whereby normal-appearing lung tissues are hypo-lucent and characterized as emphysema.

Sarah Gerard, PhD
Title/Position
Postdoctoral Research Fellow
Pulmonary vasculature patterns measured in high-resolution computed tomography (CT) images provide valuable features for characterizing lung diseases. The pulmonary vasculature consists of arterial and veinous subtrees which may be affected differently by different lung diseases. Artery and vein separation in CT is a challenging task due to similar intensity, close proximity, small size, and complex branching structure. The main focus of my research is to develop novel deep learning tools to automatically segment the vasculature and separate the vasculature into the arterial and veinous subtrees. These tools will be used to analyze large datasets of CT and dual-energy CT images from multi-institutional clinical trials and develop novel phenotypes of vasculature patterns in pulmonary diseases such as COPD.
Frank Li
Title/Position
Graduate Research Assistant
Mentor: Dr. Ching-Long Lin
My research is mainly about discovering potential phenotypes of lung diseases or subgroups of patients with lung diseases, and then simulating airflow different diseased lungs. We apply digital image processing, deep learning techniques, and big data analytics on cohorts with large amount of computed tomography (CT) images to search for possible phenotypes or subgroups. With computational fluid dynamics (CFD), we are able to simulate the airflow in the lungs, hoping to provide guidance for the doctors to customize treatments for different phenotypes or subgroups and help the patients to better manage their disease. The topics that we are currently working on are Chronic Obstructive Pulmonary Disease (COPD) and Humidifier Disinfectant-associated Lung Injuries (HDLI).

Sean Mullan
Title/Position
Graduate Research Assistant
Mentor: Dr. Milan Sonka
Deep learning can be a powerful tool, but the inherent “black box” nature of these methods makes it significantly more difficult to build the trust necessary to make the translation from research to clinical practice. My research focuses on developing explainable deep learning techniques for the segmentation and classification of 3 and 4-D pulmonary CT images. The generated explanations can provide the evidence and clarification necessary to help clinicians better understand and validate the decisions made by these models. As a further step, we are also researching the use of these generated explanations for anomaly detection. By detecting regions of CT images that cause inconsistent explanations, we can automatically detect abnormalities such as lung nodules or pneumothorax.

Carley Stewart
Mucociliary transport (MCT) is an important innate defense mechanism of the airways. Mucus traps particulate matter that enters the lung, while ciliated cells propel mucus out of the airways. Failure of this defense is implicated in many airway diseases including Cystic Fibrosis (CF). The goal of my research is to develop an imaging assay to measure MCT in healthy and CF disease states. I utilize dynamic PET imaging to capture MCT overtime and apply imaging processing techniques to quantify the movement and identify differences between CF and healthy subjects.